The Evolution of the Data Scientist
- Taru Peters-Naug
- Oct 6, 2023
- 3 min read
Oh so you know python right? Jupyter notebooks? 5 years ago the answer was of course, but no more! The face of AI and data science is changing, and has seen a significant shift in the last few years. We hear buzzwords come and go, but the key for a successful AI practice and practitioner is driving value.
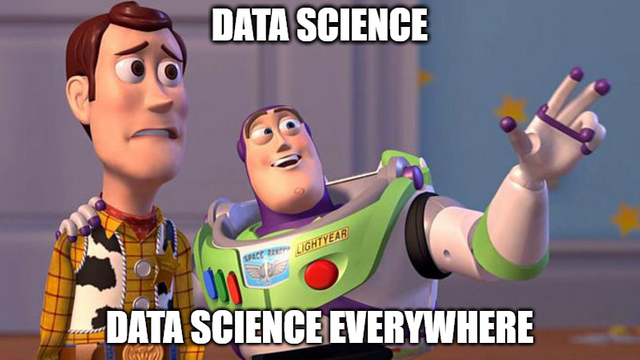
As we’ve understood the world more and more over the last few years we have ended up with a plethora of roles in the space, from Data Scientists, Machine Learning Engineers, AI Ops, AI Officers and my favourite Decision Scientists. In this article I’ll cover what I believe makes up the core skills of a great AI practitioner and how its changed over the years.
The changing landscape
Lets start with the obvious. Data is IN at the moment, everyone wants to be data-driven, use big data and the biggest carrot of all, AI. For the last 5 or so years we’ve seen technologies develop, understanding develop and interesting use cases popping up everywhere. At the same time we’ve seen what used to be a huge problems become bread and butter when it comes to AI. Things like FaceID, AI driven fraud detection and ____ are seen throughout the market now where they would have been out of reach just 10 years ago. The field has accelerated very quickly, what used to be reserved to research instutions and cutting edge technologists is starting to be available to all.
Tools Tools Tools
AI tools are everywhere at the moment. Starting from the building blocks; libraries, open-source models and libraries, but more importantly the development of AI platforms and Code-Free AI tools. The mystic world that was AI is becoming more and more accessible for a variety of skillsets.
Has technology replaced me?
The very question that everyone asks of AI, is now being asked of the people creating and working with it. If we are automating and enhancing everything, why not ourselves! This is where the crux of what makes up the best data scientists hasn’t changed and will not change going forward.
DRIVE VALUE!
This is where we start to come to the pointy end of what makes separates the good from the great. Lets be blunt, no-one outside of our geeky peers cares about your code. The rest of the world cares about the result.
I break this down into 3 key tenants which you need to satisfy for stakeholders.
1. Is the prediction / result correct and what I wanted
2. Will it always be correct
3. How do I know how the decision was made and explain it
The most effective professionals in this space UNDERSTAND THE PROBLEM. If I was to pick a perfect data scientist for a project, it would be someone with a tiny bit of data science knowledge but a lot of knowledge around the problem, the customers and the business context.
What makes takes you from good to great? Translating AI into real-world problems: AI practitioners need to be able to translate AI concepts and models into real-world problems. This means being able to identify problems that can be solved with AI, and to develop AI solutions that meet the needs of users.
Deployment
Once an AI model is developed and trained, it needs to be deployed to production so that it can be used to solve real-world problems. This process is complex and challenging, and it is important to have a good understanding of the operational aspects of AI.
Modern AI practitioners need to be able to deploy and monitor AI models in production environments, as well as integrate them with existing systems and processes. Additionally, AI practitioners need to be aware of the ethical implications of AI and how to deploy AI models in a responsible way.
The new age “data scientist”
Is a problem-solver who gets the job done. They know how to use AI to solve real-world problems, and they’re good at communicating with both technical and non-technical audiences. Plus, they’re aware of the ethical implications of AI and how to use it responsibly.
I’d summarise the perfect skillset as:
AI basics: Understand the basics of AI, including machine learning, deep learning, and natural language processing.
Real-world problems: Translate AI concepts and models into real-world problems.
Deploy: Deploy and monitor AI models in production environments.
Integrate: AI models with existing systems and processes.
Ethics: Be aware, understand and champion the ethical implications of AI and how to use it responsibly.
We as data scientists are still in high demand, however the world is constantly evolving, as much as we push the evolution we need to evolve with it. Here’s too the next 5 years being just as crazy as the last!
Comentarios